What is Data Observability? Unlock Its Potential for Success
Businesses use data for insights to fuel marketing campaigns, product development, and more. However, simply having a mountain of data isn't enough. You must ensure the data you use is accurate, complete, and trustworthy. This is where data observability enters the picture.
Data observability is a collection of practices that provide a thorough understanding of your data's health. Think of it as a transparent lens into the inner workings of your data, enabling you to identify and resolve any issues before they affect crucial decisions.
This article explores the concept of data observability, exploring its pillars and the immense benefits it offers.
What is Data Observability?
Data observability is the practice of continuously monitoring and understanding the health of your data across its entire lifecycle. This includes everything from data sources and pipelines to the transformation processes and the final destination where the data is used.
Data Observability vs. Traditional Monitoring
Data observability offers a significant advantage over traditional data monitoring methods:
- Focus: Traditional monitoring often revolves around tracking system health and performance metrics such as CPU usage or uptime. While this approach is crucial for ensuring the stability of systems, it primarily addresses the operational aspects rather than the data's journey itself. This reactive approach identifies issues only after they occur, potentially leading to data quality degradation or application failures. In contrast, data observability takes a broader view, encompassing system health and the entire data journey from its source to its consumption.
- Insights: Data observability provides deeper insights beyond simple system health. It delves into quality, lineage, and overall trustworthiness. This allows for proactively identifying potential problems before they impact data quality or other applications.
For instance, through data observability, you can detect missing values, data inconsistencies, or schema changes that may otherwise go unnoticed until they create significant issues.
Key Aspects of Data Observability
Data observability monitors several key aspects to ensure data health:
- Data Quality: This involves assessing the data's accuracy, completeness, and consistency. Data observability tools can help you identify missing values, outliers, or inconsistencies that could skew analysis or lead to erroneous conclusions. Additionally, you can monitor for schema changes that might inadvertently break data pipelines or applications.
- Pipeline Performance: Monitoring data pipelines ensures they function efficiently and deliver data on time and within expected volumes. It lets you track pipeline execution times, identify bottlenecks, and detect errors that might prevent data from being delivered on schedule. This allows proactive intervention to maintain optimal pipeline performance and avoid data latency issues.
- Data Lineage: Tracking the origin, transformations, and movement of data helps you understand its history and identify potential issues. Data observability tools allow you to map out the data lineage, showing how data flows through various systems and undergoes transformations. This lineage information is crucial for understanding the impact of data changes and troubleshooting issues that may arise downstream.
- Schema Changes: Continuously checking schema changes ensures data consistency and prevents unexpected impacts. It can facilitate tracking modifications to schemas and alerting data engineers or analysts of potential issues. This allows for proactive adjustments to data pipelines or applications to retain data integrity and prevent disruptions in subsequent processes.
What are the Five Pillars of Data Observability?
Data observability relies on a core set of pillars that offer a complete view of your data's health. These pillars act as key indicators for ensuring the quality and trustworthiness of information flowing through your systems. Here's a breakdown of these fundamental pillars:
Freshness
Freshness focuses on understanding how up-to-date your data is. Fresh data reflects the latest state, ensuring decisions are based on current information.
For instance, a sales report relying on real-time customer data for inventory management. Outdated data shows inaccurate stock levels, leading to missed sales or overstocking.
Distribution
Distribution focuses on the completeness of your data. It ensures data is available across systems or available in destinations without any errors
For example, a marketing campaign analyzes customer demographics for targeted advertising. Missing data points like zip codes or income brackets could lead to an inaccurate understanding of the target audience, resulting in ineffective campaigns.
Volume
Volume refers to the quantity of data being ingested and processed. Unexpected fluctuations might indicate data pipeline issues or external factors affecting data collection.
For instance, a financial institution monitors transaction data to detect fraudulent activity. A sudden drop in data volume might signify a system outage or a potential attempt to manipulate the data stream, hindering the ability to identify fraudulent transactions.
Schema
Schema refers to the structure and organization of your data. A consistent schema guarantees that the data remains uniform throughout the pipeline to avoid errors and misreadings.
For example, customer data collected from multiple sources might have slightly different phone numbers or address formatting. Inconsistent schema can lead to data parsing errors and integration problems, jeopardizing the accuracy of the combined data set.
Lineage
Understanding data lineage allows you to trace errors back to their source within the data pipeline. This streamlines troubleshooting and identifies the root cause of issues quickly
For example, imagine a data pipeline aggregating sales data from multiple stores. Tracking data lineage makes it easier to pinpoint the source of inconsistencies or errors in the aggregated data, which can impact accurate sales analysis and decision-making.
What are the Benefits of Data Observability?
Data observability offers many advantages for your organization if it relies on data for decision-making. Let’s explore some benefits:
Improved Data Quality and Trust
Through data observability, you can constantly monitor data for errors and inconsistencies. By identifying issues like missing values, schema violations, or data outliers, you can address them proactively. This ongoing process significantly improves data quality, ensuring a reliable foundation for analysis and decision-making. Trust in the data increases as you have greater confidence in its accuracy and completeness.
Faster Issue Identification and Resolution
Data observability, with its proactive approach, allows for the early detection of potential problems. Through continuous data pipelines and health metrics monitoring it can help you identify anomalies and potential issues before they escalate. This enables you to act swiftly, minimizing the impact of data problems and ensuring timely resolution.
Increased Confidence in Decisions
When data quality is questionable, you risk making decisions based on inaccurate or incomplete information. Data observability helps ensure the data used for analysis is reliable and trustworthy. This allows you as decision-makers to have greater confidence in the insights derived from data, leading to more effective strategies and improved outcomes.
Streamlined Data Governance
Data observability practices promote data ownership and accountability within an organization. By providing clear visibility into the data lifecycle, data observability tools help identify who is responsible for different data assets. This facilitates better data governance by ensuring proper data ownership and adherence to compliance regulations.
Enhanced Operational Efficiency
Data pipelines are the workhorses constantly ingesting and processing data. Data observability helps you to identify bottlenecks within these pipelines, allowing for optimization and improved processing speeds. This enables you to promptly access critical data for analysis and reporting, ultimately enhancing operational efficiency across your organization.
What are the Challenges of Data Observability?
The implementation of data observability frameworks presents several significant hurdles. Here's a breakdown of these challenges:
Tool Selection and Integration
Many tools are available, each with its own strengths and weaknesses. Choosing the right ones requires careful consideration of your company's specific needs. Factors like the amount of data you handle, the variety of data sources you use, and your budget play a role in tool selection.
Data Quality Ownership and Accountability
Effective data observability relies on clearly defined ownership and accountability for data quality. This can be difficult when multiple teams or departments use the same data sets. To avoid confusion, it is crucial to define who is responsible for maintaining the health and accuracy of specific data sets.
Securing Skilled Personnel
Maintaining a framework necessitates a team with specialized skills. These personnel need expertise in data pipelines, data quality concepts, and the specific tools you've chosen. They should be able to manage data movement efficiently, understand principles, and use tools to monitor, alert, and troubleshoot data issues. Finding and retaining these skilled professionals can be challenging.
Managing Diverse Data Sources
Businesses collect data from various sources, such as databases, applications, sensors, and social media. Data observability solutions must be adaptable and handle these diverse data formats effectively. Before you can leverage data observability, you need a unified view of your data. This is where data integration tools like Airbyte simplify the process of bringing data from all your disparate sources into a central location, like a data warehouse or data lake.
Introducing Airbyte into Your Data Observability Practices
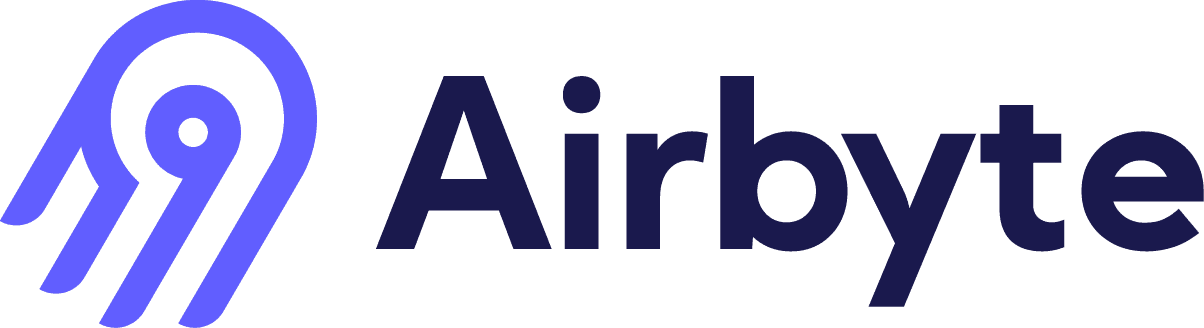
Airbyte, a data integration tool, can play a critical role by providing transparency into data pipelines and enabling integration with modern data stacks. This combination empowers you to monitor data flow health, identify potential issues early, and ensure high-quality data reaches your analytics tools.
Here’s how Airbyte can help you with data observability:
- Pre-built Connectors: Airbyte has a library of 350+ pre-built connectors that seamlessly integrate with a wide range of data sources. The extensive support eliminates the need for custom development in many cases, allowing for quicker setup and easier data pipeline building.
- Change Data Capture (CDC): This functionality in Airbyte enables monitoring of data updates by tracking incremental changes in datasets. This ensures immediate visibility into any alterations to the data, enhancing data observability.
- Connector Development Kit (CDK): For less common data sources or those with unique requirements, Airbyte offers a Connector Development Kit (CDK). This allows you to build custom connectors that extend Airbyte's functionality and integrate with even niche data sources. In other words, this flexibility ensures that your data observability framework can adapt and evolve alongside your specific data needs, even when dealing with non-standard data sources.
- Monitoring and Alerting Capabilities: Airbyte enables you to integrate with monitoring platforms like Datadog. This lets you monitor the health and performance of your data pipelines, ensuring timely detection of issues.
- Integration with Data Orchestration Tools: You can integrate Airbyte with popular data orchestration tools like Airflow, Prefect, and Dagster. This integration allows you to schedule and manage your pipelines within your existing workflow environment, streamlining data observability tasks.
- PyAirbyte: Airbyte offers a Python library called PyAirbyte. The library allows developers to interact with Airbyte programmatically from within Python code. This functionality can be particularly useful for building custom data transformations or scripting tasks directly in your data pipelines.
Conclusion
With a focus on quality, businesses can avoid decisions based on faulty data. Data observability offers a critical path forward. This approach involves continuous monitoring of data health. By actively identifying issues, you can prevent them from causing disruptions. The forward-thinking strategy leads to cleaner data, faster resolutions, and more confident choices fueled by reliable information.